AI-Driven Predictive Maintenance: Manufacturing’s Next Digital Frontier
With better connectivity and big data collection, artificial intelligence is set to power up intelligent asset maintenance and bring operational optimization across factory floors.
How Is AI Used in Manufacturing?
Manufacturers have only begun to capitalize on artificial intelligence (AI) and machine learning capabilities on the factory floor. Today, AI’s key roles in manufacturing expand beyond robotics and automation – they now include driving overall equipment effectiveness (OEE), optimizing processes and creating a pivotal role in predictive maintenance.
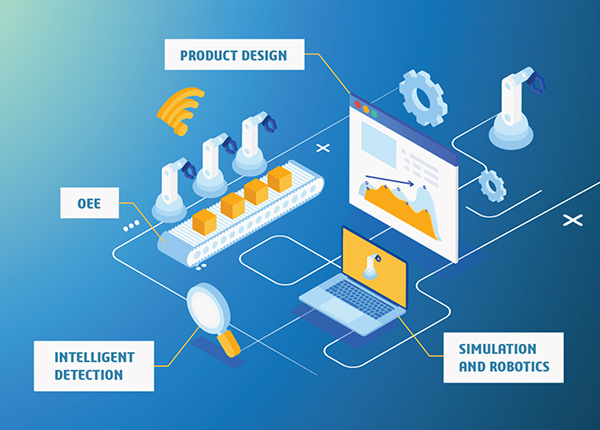
How Is AI Used in Manufacturing?
AI provides in-depth analysis to achieve overall equipment effectiveness (OEE) optimization. Used to monitor and detect missing materials or quality issues, AI enables automatic failure notice alerts in production control, allowing manufacturers to reduce the impact of such failures and maintain high-performance assets.
AI automates defect detection through intelligent vision systems and video analytics technology. A vision system quickly identifies misaligned parts and missing or wrong components with minimal human error. This dramatically improves product quality.
In warehouse management, vision systems and video analytics detect available spaces and damaged packaging on shelves while helping manufacturers determine optimal equipment handling path and timing for production materials.
AI-enabled simulation and robotics are used to identify optimal movement path (materials movement) and sequence (production steps) before the actual implementation. This is an effective way to minimize manufacturing scraps, avert accidents and improve maintenance. AI’s simulation to reach optimal balanced heat, light and air, lead to better energy management, too.
AI technology captures and tracks design data over time, allowing engineers to deliver innovative product designs and reduce test times while enabling more concrete insights from customer demands.
McKinsey reports that the greatest value from AI can be created by using it for predictive maintenance, generating about $0.5 trillion to $0.7 trillion across the world’s business in potential value impact. AI’s goal in predictive maintenance is simple: Analyze massive real-time data speedily to predict assets failure intelligently, so that manufacturers can keep their mission-critical assets running at peak performance.
Make AI a Key Part of Your Factory Floor
Learn how AI-driven predictive maintenance optimize and deliver efficiency across the factory floor.
Identify Obstacles in AI-Driven Predictive Maintenance
Although manufacturers are set to gain significantly from AI, they must address potential challenges that come with incorporating AI in predictive maintenance.
The Potential Challenges of Adopting AI-Driven Predictive Maintenance
Data Security
The use of internet of things (IoT) devices to collect data on the cloud exposes data to security issues such as unauthorized access and unauthenticated manipulation.
An enhanced cybersecurity system needs to be deployed to safeguard intellectual property rights and sensitive customer data.
Data Integration and Aggregation
Data collected are usually unstructured, time-based, high-volume and in different formats.
The right platform with storage capabilities and advanced analytics are required for efficient data integration and aggregation to generate meaningful insights.
Usable Historical Data
Large quantities of historical data are essential for effective predictive maintenance.
Collecting historical data requires substantial time and effort to identify data types, capture and build suitable algorithms and allow machine learning to work.
Machine Readiness and Interoperability
Without the correct number of sensors and actuators in the precise location, the data quality and communication will be affected.
The lack of a single platform to integrate data from environmental sources and other systems will hamper effective execution.
Cost and Expertise
Higher upfront financial investment on sensors, software and necessary equipment may deter adoption.
Getting the right skills set is a challenge too. Often expertise in managing multiple different technologies is crucial to driving decisions based on the data collected.
AI is required for effective predictive maintenance. With it, manufacturers can evaluate multiple inputs like usage, vibration and noise directly from the machine, compare that to historical events and forecast when a machine failure is likely to occur. It then schedules the appropriate maintenance activities at the last reasonable moment before the expected failure.
Stay Ahead With Intelligent Solutions
On the 3DEXPERIENCE platform, manufacturers can overcome challenges associated with adopting AI-driven predictive maintenance. They can experience the full potential of AI in predictive maintenance by incorporating these key applications on the platform:
- The Rise of Edge Computing
- The Virtual Twin Advantage
- Collaborative and Unified Platform
The Rise of Edge Computing
Edge computing brings intelligence and processing power a step closer − to the actual source device for faster and deeper insights. Its revolutionary approach in maintenance with AI improves communication on infinite endpoints to maximize data collection and place sensors in strategic areas for optimal monitoring. Quick detection and rectification of anomalies are processed and executed at the sensor itself. This intelligent capability averts sudden stoppage, significantly reduces latency to drive business decisions faster and more accurately while avoiding expensive cloud transmission costs.
The Virtual Twin Advantage
Virtual twin technology enables manufacturers to use simulation to determine the type and amount of data to collect and the flow of data set to create an effective predictive maintenance model. Manufacturers can plan, model and test sensors and perform motion planning of factory floor layout to eliminate any environmental risk factors that could cause maintenance issues – all without incurring the time and money costs of an actual implementation. Manufacturers gain a 3D view of plant operations with activity history to determine factors contributing to an incident and respond accordingly.
Collaborative and Unified Platform
The platform simplifies the user experience by enhancing collaboration through the four pillars of predictive maintenance (monitoring, diagnosis, recommendation and execution). It resolves data challenges by optimizing data preparation and ingestion through intelligent analytics and broad integration functionalities. On the platform, data is collected, stored and processed seamlessly for best utilization. With end-to-end visibility across multiple divisions, manufacturers can process various structured or unstructured data sources to become one shared source of truth.
Read our top articles on Operational Optimization
Explore our Industry Solution Experiences
Learn more about how our industry solutions can help you to achieve your professional ambition and business objectives